As the power and influence of artificial intelligence continue to grow, it becomes increasingly crucial to address the pressing issue of gender bias embedded within generative AI systems, raising important ethical questions and necessitating a comprehensive exploration of its ramifications.
I was walking the other day with an actress-friend who auditions for a wide variety of roles in New York City. She told me how she had just tried out for a police officer role in a major crime drama. As part of her background research before each tryout, she googles what a female in that role would wear so she can look the part when she auditions. When she googled “female police officer,” she got a few hits of professional-looking women in the classic cop shirt tie + hat combo, followed by a series of images of women wearing sexy police Halloween costumes. She told me she has had similar role-related search experiences going back for many years now. As mothers of 16-year-old daughters at a leading New York City STEM school, we commiserated about the continued lack of aspirational representation in our most heavily relied-upon algorithms.
We got to talking about this because I was sharing my excitement about gender bias in generative AI and for companies such as ChatGPT that might transform how we approach innovation and how we work in many other fields. Innovation is richer and more productive when you have divergent and broad stimuli, and generative AI can grab vast amounts of data and quickly synthesize results. For example, a simple query juxtaposing “NASA” and “Nike” produced some remarkably good starting points for thinking about new concepts for sneakers.
But as we’ve been experimenting with using models including ChatGPT, Dall-E2 and Rationale for innovation, I’ve also been trying to provoke them so I could gain a first-hand understanding of the extent to which they are gender biased.
Here’s what I found:
When I tried DALL·E 2 (OpenAI’s new system “that can create realistic images and art from a description in natural language”), I got back results that would be dismayingly familiar to my actress friend. For instance, when I searched for “CEO”, I got three images of Caucasian middle-aged men and one young Hispanic professional woman. In my marketing days, we called this “your strategy is showing” – meaning you’re being so obvious that it’s hard to be believed. (Were the results here generated entirely by AI, or were they adjusted by humans to conceal gender bias in generative AI?) So while I appreciated that a woman was represented, I was still disappointed.
Similarly, when I asked ChatGPT what careers are best for women, I got first the usual human-scripted disclaimers (“Women are equally capable of pursuing any career they desire and should have the same opportunities as men to achieve success in their chosen field”) and then this ranking:
- Healthcare
- Education
- Business
- Social work
ChatGPT ranked the best careers for men as:
- Engineering
- Information technology
- Business
- Science
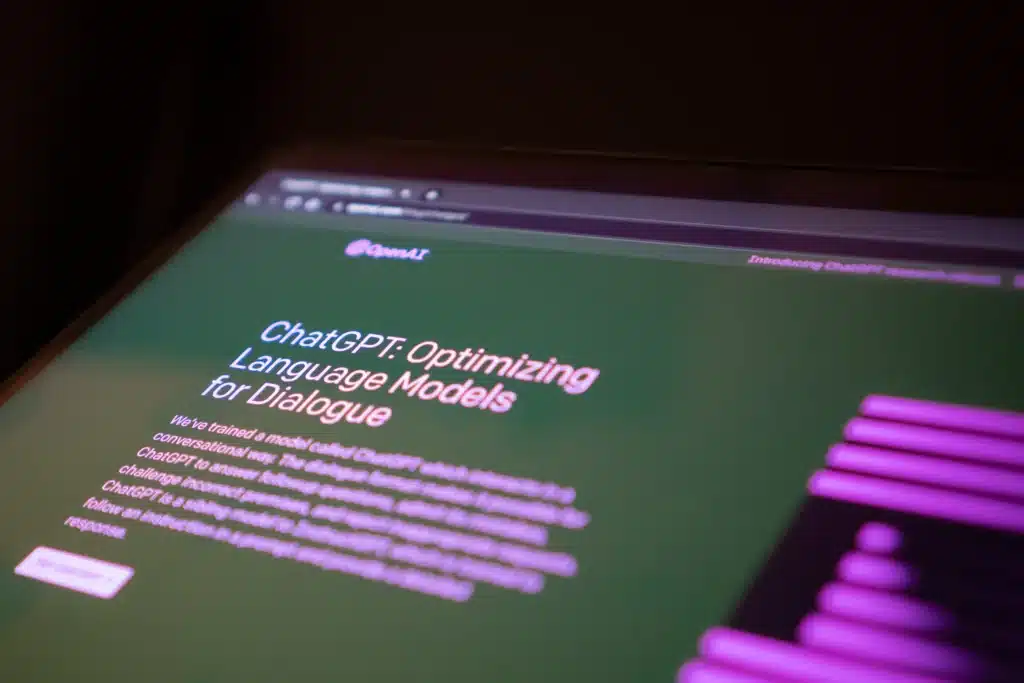
When I then queried ChatGPT to determine the average salaries for these exact categories and averaged the results, it informed me that men will make 22% more if they pursue their suggested careers, as compared to women who pursue their respective ChatGPT-suggested careers. Another ChatGPT query tells me that this is six percentage points more of a “gender pay gap” for women vs. men than the 16% gap reported by the World Economic Forum in 2021. Even more disappointing.
As I see it, two issues lead to this type of bias:
First, most of the “training” for most of AI is done on databases that reflect our historical biases. As Meredith Broussard, author of Artificial Unintelligence: How Computers Misunderstand the World puts it “Certain 1950s ideas about gender are actually still embedded in our database systems.” More generally, all the bad ideas about gender published in earlier centuries are still part of the present record, thanks to vast online libraries such as Project Gutenberg and Google Books. We can’t erase the gender-biased culture we’ve collectively inherited, although it is possible to append disclaimers for a few of the most egregious artifacts. Given this, incorporating more of a recency bias into our algorithms, or another more intentional targeting for AI training, might be strongly preferable to giving so much weight to the whole historical record.
The second issue is one of representation. When I look at the list of founders of OpenAI, it’s not unlike any other Silicon Valley unicorn. But it’s still freshly troubling:
- Greg Brockman (Founder & CTO)
- Samuel Altman (CEO)
- Elon Musk (Founder)
- Ilya Sutskever (Founder and cowboy from the looks of his Linkedin)
- Wojciech Zaremba (Founder)
- John Schulman (Founder)
Six men working together to make sure AI is “accessible to as many people as possible” and that its “technologies are used in a responsible and ethical manner.””Further investigation shows the current OpenAI executive team is: 21% female and 79% male. (Mira Murati has played a leadership role in developing the ChatGPT application of OpenAI, but I’m concerned the attempts to put her forward so often as the pubic face of the product are at least in part “genderwashing.”) More generally, a 2020 World Economic Forum report on gender parity suggests women account for only 26% of data and AI positions in the workforce.
At a recent (Feb 14) high-profile generative AI conference in SF, only 2 of the 18 speakers were women. No women appeared on any of the substantive panels; instead, the two female participants were featured in a “spoken word” performance and marketing talk. The issue of gender equality and AI did not show up on the agenda. Further, every participating founder/CEO – and every single founder/CEO of a generative AI company that I could find anywhere – is male (Craiyon, Copy.ai, Grammarly, Jasper, Krisp, Stability AI, Stable Diffusion, TLDr, to name a few…)
I worry that generative AI gender biases – and the fraternity that is now deciding how to configure these new tech systems – will undermine the broader efforts to address gender gaps in the workforce. STEM education has, very slowly, been getting more inclusive. At my daughter’s STEM high school, for example, they now have the “FeMaidens” – a robotics team of girls and gender minorities and it’s one of the best in the city. Beyond formal education, there are many excellent mentoring organizations such as Girls Who Code. But gender gaps remain: for example, according to the latest data, only 22% of engineering students are female.
Is it ethical for young girls like my 16-year-old daughter to rely on 1950s stereotypes telling her that, while it’s her personal choice, traditionally, the best careers for her would earn her at least 22% less, on average, than a man? If we continue to tell our young girls that the most appropriate careers for them are NOT in Science, Engineering and IT, we will never solve the gender representation issue.
Equal (or maybe even starting with “some”) participation in who gets to configure generative AI would be an important first step to ensure more enlightened representation in these powerful new tools. As we celebrate International Women’s Day, a day that is about celebrating gender equality, advancing gender equity and reducing bias, my hope for the 16-year-olds out there is that we work to solve this challenge as aggressively as we work to develop the technology. Because I’d like my daughter to actually make 22% more than a man.
Click here for more on International Women’s Day.
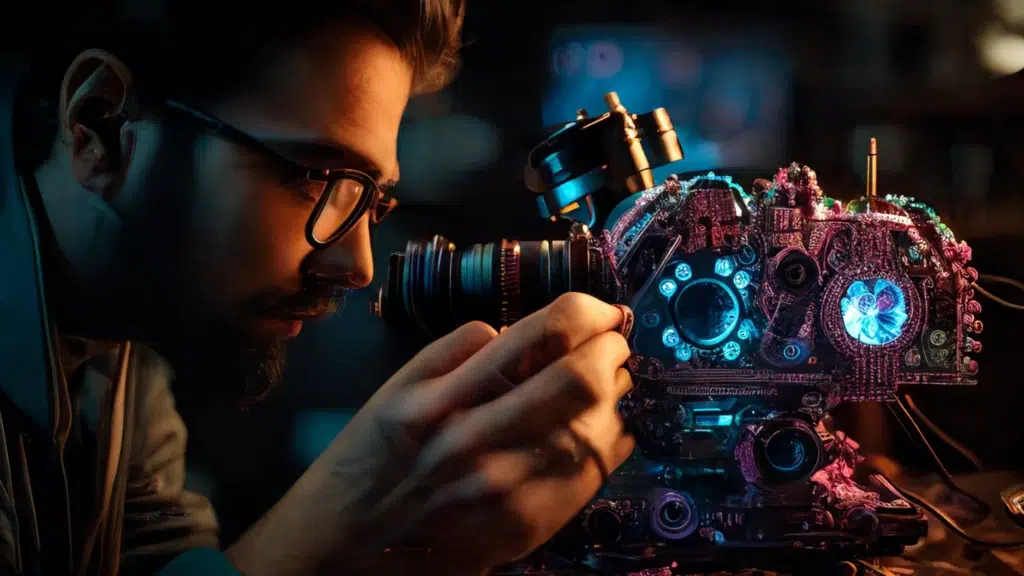